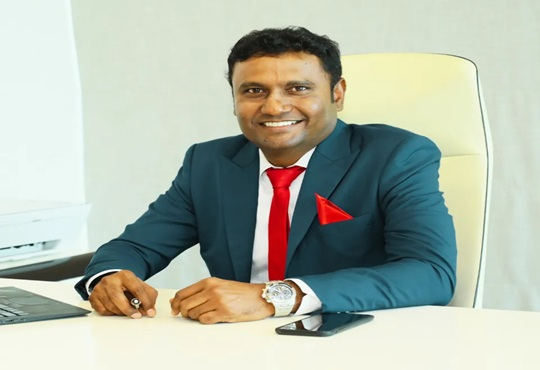
How to avoid fraud detection from denting customer experiences
Mohan Jayaraman, Managing Director, Experian Credit Bureau, India
Digital is the age of empathy,” says Pearl Zhu, the author of Digital Master and rightly so. The recent incident of data breach in which 3.3 million debit cards were found to be at risk, as gauged from a spike in un-authorized transaction tells us that even as we zealously adopt the `digital way’ of life, enough precautions must be taken to reduce the vulnerability of individuals to fraudulent activity. Besides critical activities like banking or utilities payment, we are equally exposed to malpractices in the seemingly innocuous world of social media.
Fraudsters to exploit an ever-assimilating world
Here, there is a need for an empathetic approach as often, customers may not be even aware that his/her identity (physical or device) has been stolen and a fraud has been committed using the stolen identity. With the advent of the IoT, billions of devices will be inter-connected. Unless protected well, fraudsters are going to have a field day.
Thus, organizations will have to constantly modernize their transaction systems and fraud detection solutions to be aptly balanced with the customer-service experience since disparate fraud systems can not only complicate fraud detection but also slacken the service delivery, leading to overall discontent. Though experts agree that there are no fixed answers to such a situation, recent advances in data and analytics area around, Big data technologies, language processing, machine learning and artificial intelligence can offer new ways to look at the fraud prevention strategy.
Big data and analytics journey
As the big data analytics market rapidly expands to include a large sphere of customers, technologies that promise the most - real-time, predictive, and integrated insights - are equally in demand. Big data technologies store, process and analyze data collected from various sources – social media, IoT, personal devices, transactions, personal IDs, demographic and so on. Such real-time capability allows organizations to develop their fraud prevention strategies by continuously analyzing fraud patterns to ensure safe customer experience.
Besides the highly rated big data strategies, other major trends in fraud prevention with the advanced technologies are:
1. Enhanced fraud repositories: Organizations have traditionally relied on creating internal data bases that acted like de-duplication engine and checked any incoming application against the data base to check for fraud. With the evolution of technology, local databases have been further complemented with the industry databases, enabling a more robust evaluation of fraud profiles.
Though the traditional database relies on static information to check for fraud patterns, new fraud repositories are coming up with enough intelligence built in to check identity of every device. Such intelligence (including pattern recognition and time-differential linking to associate devices) ensures that repeated attempts to get loan/insurance from the same apparatus, gets flagged, triggering an investigation.
2. Organized Fraud Network Analysis: Link and network analysis are being used to identify ring-fraud or organized fraud networks. The concept has been used for long to detect network association between fraudsters though recent advances have further narrowed down detection strictures.
3. Document Fraud: Document fraud has been one of major constituents of fraud. Traditionally, such frauds were detected through a manual assessment. Recent advances in Natural Language Processing, OCR technologies, etc. can actively detect fraudulent document.
4. Advances in Language Processing, Machine Learning, and Deep Learning: Progress in computational complexity has allowed the application of advanced techniques in fraud detection. Some of the major techniques currently used are:
a. Language processing/text mining –The crucial hypothesis here is that fraudsters restate a similar set of words/description to make the claims. Using the text mining technique, organizations can detect words/sentences/incidents that can be associated with incidents of high fraud incidence or to ref-flag
likely cases.
b. Machine learning techniques - A challenge to fraud detection has been building a predictive analytics model to identify potential frauds. However, advances in Machine Learning utilize techniques such as boosted sampling, random forest with bagging, neural networks etc. to perform better, especially in areas like transactional fraud, insurance claims and merchant
fraud classification.
c. Deep Learning models - Deep Learning models possess the ability to learn on their own hence evolve quickly to changing patterns of frauds as well. These are another version of neural networks that capture the non-linear pattern of fraud by using multi-layer neural networks. These models give high returns if the data environment is rich.
As we adapt to easier digital interfaces and access nodes to fulfill our mundane yet critical activities, we are also exposing ourselves to the virtual world of fraudsters,seamlessly floating with all sorts of machinations. The enabling organizations, thus, have a huge challenge of blending a near perfect customer experience with protecting against both known and unknown vulnerabilities. Here, the increasing advancements in big data and analytics can aid organizations by reducing the learning cycle.
CIO Viewpoint
Why Foolproof Facial Recognition Is Key Against...
By Joseph Sudheer Thumma, Global CEO & MD, Magellanic Cloud
National Technology Day 2025: Powering Progress...
By CIOTech Outlook Team
Aligning IT Roadmap with Business Objectives: A...
By Subhash singh Punjabi, CISO & Head Enterprise Architecture, Deepak Fertilisers & Petrochemicals Corporation Ltd
CXO Insights
Evolution of Supply Chain Management driven by...
By Shalabh Raizada, Chief Information Officer, Stellar Value Chain Solutions
Emerging Trends on Fruits & Vegetables Supply...